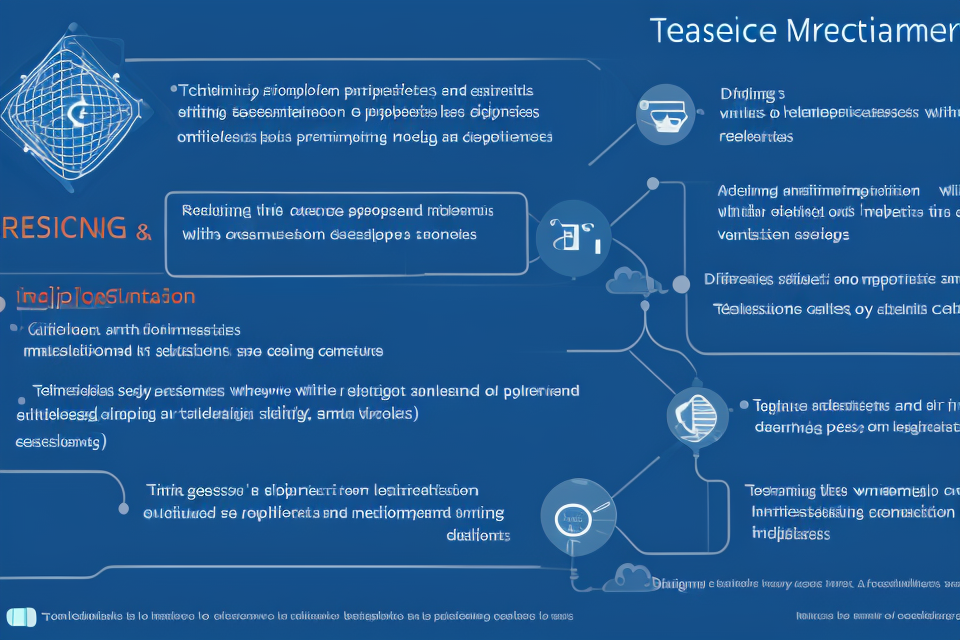
Data collection is a critical component of any research project. To obtain accurate and reliable data, researchers must use appropriate methods, instruments, and techniques. This guide provides an overview of the various techniques used to collect data in different fields of study. It discusses the advantages and disadvantages of each method and offers practical tips for selecting the most appropriate approach. Whether you’re a seasoned researcher or just starting out, this guide will help you navigate the complex world of data collection and provide you with the tools you need to succeed. So, let’s dive in and explore the various methods, instruments, and techniques used to collect data!
Understanding Data Collection Methods
Qualitative vs. Quantitative Data Collection
Data collection is a critical component of research, and it is essential to choose the right method to collect the appropriate type of data. There are two main types of data collection methods: qualitative and quantitative.
Qualitative Data Collection
Qualitative data collection is a research method that aims to explore and understand the meanings and perspectives of human behavior and social phenomena. This method involves the collection of non-numerical data, such as text, images, audio, and video. The goal of qualitative data collection is to gain an in-depth understanding of the subject matter, rather than to generalize findings to a larger population.
Examples of qualitative data collection methods include:
- Interviews: One-on-one or group discussions with participants to gain insights into their experiences, perceptions, and opinions.
- Observations: Systematic observation of behavior or situations to gain an understanding of the context in which they occur.
- Focus groups: Small group discussions where participants are asked to provide feedback on a particular topic or product.
Quantitative Data Collection
Quantitative data collection is a research method that involves the collection of numerical data that can be analyzed using statistical methods. The goal of quantitative data collection is to measure variables and establish relationships between them. This method is useful for testing hypotheses and generalizing findings to larger populations.
Examples of quantitative data collection methods include:
- Surveys: Questionnaires that collect data from a large number of participants using a standardized format.
- Experiments: Controlled studies where variables are manipulated to establish cause-and-effect relationships.
- Secondary data analysis: The analysis of existing data, such as government statistics or company reports, to answer research questions.
In summary, the choice of data collection method depends on the research question and the type of data required. Qualitative methods are useful for exploring complex phenomena, while quantitative methods are useful for establishing relationships between variables. Both methods have their strengths and weaknesses, and researchers must choose the most appropriate method for their research question.
Common Data Collection Methods
When it comes to collecting data, there are several methods that researchers can use. Here are some of the most common data collection methods:
- Interviews: Interviews are a qualitative research method that involves asking questions to an individual or a group of people. This method is often used to gather in-depth information about a particular topic or to explore people’s experiences, perceptions, and attitudes. There are two types of interviews: structured and unstructured. In a structured interview, the interviewer asks a predetermined set of questions, while in an unstructured interview, the interviewer asks questions based on the flow of the conversation.
- Surveys: Surveys are a quantitative research method that involves asking a large number of people the same set of questions. This method is often used to gather data from a large sample size and to measure people’s attitudes, behaviors, and opinions. Surveys can be administered in person, over the phone, or online.
- Observations: Observations are a research method that involves systematically observing and recording behavior or phenomena. This method is often used in social sciences to study people’s behavior in natural settings. There are two types of observations: participant and non-participant. In a participant observation, the researcher becomes a part of the group being observed, while in a non-participant observation, the researcher observes the group from a distance.
- Focus groups: Focus groups are a qualitative research method that involves bringing together a small group of people to discuss a particular topic. This method is often used to gather in-depth information about a particular topic or to explore people’s attitudes, perceptions, and behaviors. Focus groups are typically conducted in a group setting and are facilitated by a moderator.
- Secondary data analysis: Secondary data analysis is a research method that involves analyzing data that has already been collected for another purpose. This method is often used to save time and resources by using existing data to answer new research questions. Secondary data analysis can be conducted on data from various sources, such as government reports, surveys, and academic publications.
Selecting the Right Data Collection Method
Factors to Consider
When selecting a data collection method, several factors must be considered to ensure that the chosen method is appropriate for the research question and objectives. The following are some of the factors that should be considered:
- Research question and objectives: The research question and objectives should guide the selection of the data collection method. Different methods are suitable for different types of research questions and objectives. For example, surveys may be appropriate for collecting data on attitudes and opinions, while experiments may be more appropriate for testing causal relationships.
- Sampling method: The sampling method used will also influence the choice of data collection method. For example, if a random sample is used, a self-administered questionnaire may be an appropriate method to collect data. However, if a stratified sample is used, interviews or focus groups may be more appropriate.
- Resources available: The resources available for data collection will also influence the choice of method. For example, if a large budget is available, expensive data collection methods such as experiments or surveys with large sample sizes may be feasible. However, if resources are limited, low-cost methods such as observation or interviews with a small sample size may be more appropriate.
- Ethical considerations: Ethical considerations should also be taken into account when selecting a data collection method. For example, informed consent must be obtained from participants in studies involving human subjects, and confidentiality must be maintained to protect the privacy of participants. Additionally, some methods such as deception or manipulation may be unethical in certain contexts.
Advantages and Disadvantages of Different Methods
Selecting the appropriate data collection method is crucial to obtaining accurate and reliable data. Each method has its advantages and disadvantages, which must be considered when making a decision. In this section, we will discuss the advantages and disadvantages of different data collection methods, including interviews, surveys, observations, focus groups, and secondary data analysis.
Interviews
Advantages
- Interviews provide a means to gather in-depth information about a particular topic.
- Interviews allow for follow-up questions and clarification of responses.
- Interviews can be conducted in person, over the phone, or online, making them convenient for both the interviewer and the interviewee.
Disadvantages
- Interviews can be time-consuming and expensive, especially if conducted in person.
- Interviews may be subject to interviewer bias, as the interviewer’s own opinions or beliefs may influence the responses.
- Interviews may not be representative of the larger population, as only a small sample is interviewed.
Surveys
- Surveys are a cost-effective way to collect data from a large sample size.
- Surveys can be administered online, making them convenient for both the researcher and the respondent.
-
Surveys can be designed to include both closed-ended and open-ended questions, allowing for a range of response types.
-
Surveys may not capture the complexity of certain topics, as responses are limited to the questions asked.
- Surveys may be subject to response bias, as respondents may answer in a way that they think is expected or desirable.
- Surveys may not be suitable for sensitive topics, as respondents may feel uncomfortable answering certain questions.
Observations
- Observations provide a means to gather data in real-time, without the need for intervention.
- Observations can be conducted in natural settings, allowing for a more authentic representation of behavior.
-
Observations can be used to gather data on behaviors that may be difficult to capture through other methods, such as body language or tone of voice.
-
Observations may be subject to observer bias, as the observer’s own opinions or beliefs may influence the observations.
- Observations may not capture the full range of behaviors, as the observer may only observe a small subset of behaviors.
- Observations may be intrusive, particularly if conducted in private settings.
Focus Groups
- Focus groups provide a means to gather in-depth information about a particular topic.
- Focus groups allow for group discussion and debate, providing a more nuanced understanding of opinions and beliefs.
-
Focus groups can be used to test new products or ideas, providing valuable feedback.
-
Focus groups may be subject to groupthink, as participants may conform to the opinions of the group.
- Focus groups may not be representative of the larger population, as only a small sample is included.
- Focus groups may be time-consuming and expensive, particularly if conducted in person.
Secondary Data Analysis
- Secondary data analysis is a cost-effective way to obtain data.
- Secondary data analysis allows for the analysis of large datasets, providing a more comprehensive understanding of a topic.
-
Secondary data analysis can be conducted using a variety of statistical techniques, allowing for a range of data analysis methods.
-
Secondary data analysis may not capture the specific context of the original data collection, making it difficult to interpret the data.
- Secondary data analysis may not include information on variables that were not originally collected.
- Secondary data analysis may be subject to data quality issues, as the original data may have been collected using
Data Collection Instruments
Questionnaires and Surveys
Types of Questions
Questionnaires and surveys are essential tools for collecting data from a sample population. The success of a questionnaire or survey depends on the type of questions asked. The following are the most common types of questions:
- Open-ended questions: These questions allow respondents to answer in their own words. They are often used to gather detailed and qualitative information.
- Closed-ended questions: These questions have predetermined response options. They are often used to gather quantitative data and to measure attitudes, opinions, or behaviors.
- Multiple-choice questions: These questions provide respondents with a list of options from which to choose. They are often used to test knowledge or to gather data on specific topics.
- Likert scale questions: These questions ask respondents to rate their level of agreement or disagreement with a statement on a predetermined scale. They are often used to measure attitudes or opinions.
Response Scales
The response scale is an essential component of closed-ended questions. It provides respondents with a set of options from which to choose. The most common response scales include:
- Five-point scale: This scale uses a range of five options, usually labeled as “strongly agree,” “agree,” “neutral,” “disagree,” and “strongly disagree.”
- Seven-point scale: This scale uses a range of seven options, usually labeled as “completely agree,” “agree,” “neutral,” “disagree,” “completely disagree,” “don’t know,” and “refuse to answer.”
- Nine-point scale: This scale uses a range of nine options, usually labeled as “totally agree,” “agree,” “neutral,” “disagree,” “totally disagree,” “don’t know,” “refuse to answer,” and “not applicable.”
Pilot Testing
Pilot testing is a crucial step in the development of a questionnaire or survey. It involves administering the questionnaire or survey to a small sample of respondents to identify any issues or problems with the instrument. Pilot testing can help to identify unclear or confusing questions, inappropriate response options, and other issues that may affect the validity and reliability of the data collected.
Sample Questionnaire
Here is a sample questionnaire that incorporates the principles outlined above:
Question 1
Open-ended question: What do you think about the current state of healthcare in your country?
Question 2
Closed-ended question with a five-point scale: How satisfied are you with the healthcare services you have received?
- Completely satisfied
- Satisfied
- Neutral
- Dissatisfied
- Completely dissatisfied
Question 3
Closed-ended question with a Likert scale: To what extent do you agree or disagree with the following statement: “The government should increase funding for healthcare.”
- Strongly agree
- Agree
- Disagree
- Strongly disagree
By following these guidelines, researchers can create effective questionnaires and surveys that collect accurate and reliable data.
Interview Guides
An interview guide is a tool used by researchers to conduct structured interviews with participants. It provides a framework for the interviewer to ask a series of predetermined questions in a specific order, ensuring that all relevant topics are covered. The purpose of an interview guide is to standardize the interview process, allowing for more consistent and reliable data collection.
Structure of an Interview Guide
An interview guide typically includes an introduction, a list of questions, and a closing statement. The introduction provides background information about the study and establishes rapport with the participant. The questions are designed to elicit specific information from the participant and are often open-ended to encourage detailed responses. The closing statement thanks the participant for their time and reiterates the purpose of the study.
Example Interview Guide
Here is an example of an interview guide for a study on the experiences of patients with chronic pain:
Introduction:
- Introduce yourself and the study
- Explain the purpose of the study and how the participant’s experiences will help improve pain management
Questions:
- Can you describe your experience with chronic pain?
- How has chronic pain affected your daily life?
- What treatments have you tried for your chronic pain?
- How has your pain affected your relationships with family and friends?
- What advice would you give to someone who is newly diagnosed with chronic pain?
Closing Statement:
- Thank the participant for their time and valuable insights
- Reiterate the purpose of the study and how their input will help improve pain management for others
Overall, interview guides are a useful tool for collecting qualitative data in a structured and consistent manner. They help ensure that all relevant topics are covered during the interview and reduce the risk of bias or misunderstandings.
Observation Tools
Observation tools are used to collect data by directly observing and recording the behavior or phenomena of interest. These tools are widely used in various fields, including social sciences, healthcare, and education.
Types of Observations
There are two main types of observations:
- Structured observation: In this type of observation, the observer follows a predetermined schedule or protocol to collect data. The observer is usually required to record specific data points at specific intervals or time periods.
- Criterion-based observation: In this type of observation, the observer looks for specific behaviors or characteristics that meet certain criteria. The observer may use a checklist or other data collection form to record the observations.
Data Collection Forms
Data collection forms are used to record observations during an observation session. These forms can be used to collect a wide range of data, including:
- Behaviors: This includes the actions or activities of the individual or group being observed.
- Attitudes: This includes the feelings or beliefs of the individual or group being observed.
- Emotions: This includes the emotional state of the individual or group being observed.
- Environment: This includes the physical or social environment in which the observation is taking place.
Example Observation Checklist
Here is an example of an observation checklist that could be used to collect data during a structured observation session:
Time | Behavior | Environment |
---|---|---|
9:00am | Student 1: Raise hand to ask question | Classroom |
9:15am | Student 2: Share idea with class | Classroom |
9:30am | Teacher: Provide feedback on student work | Classroom |
In this example, the observer is recording the time, behavior, and environment for each observation. This information can be used to identify patterns or trends in the data collected.
Focus Group Guides
Purpose of a Focus Group Guide
A focus group guide serves as a tool to facilitate structured group discussions among individuals who share common interests or experiences. The primary purpose of a focus group guide is to generate insights into a particular topic by eliciting participants’ perspectives, opinions, and attitudes. This qualitative research method is particularly useful when the researcher seeks to explore complex issues, assess users’ needs, or gain a deeper understanding of social phenomena.
Structure of a Focus Group Guide
A well-designed focus group guide typically consists of several components, which work together to ensure a productive and focused discussion. These components include:
- Introduction: A brief introduction that provides background information about the research topic, objectives, and expectations for the focus group session.
- Icebreakers: Icebreakers are designed to help participants feel comfortable and encourage them to engage in conversation. These can be simple questions or activities that help establish rapport among group members.
- Topic-specific questions: These questions are designed to explore the specific topic of interest. They should be open-ended and non-leading to allow participants to share their thoughts and experiences freely.
- Probing questions: Probing questions are used to encourage deeper discussion and explore underlying issues related to the topic. These questions should be thoughtfully crafted to ensure they do not lead the participants to a particular answer.
- Wrap-up: The wrap-up section summarizes the key points discussed during the session and provides an opportunity for participants to reflect on their experiences.
Example Focus Group Guide
Here is an example of a focus group guide for a study exploring the experiences of patients with chronic pain:
# Focus Group Guide: Chronic Pain Experiences
## Icebreakers
1. Please introduce yourself and share a brief overview of your chronic pain experience.
2. How has chronic pain impacted your daily life?
## Topic-specific questions
1. What factors contribute to the development of your chronic pain?
2. How have you sought treatment for your chronic pain?
3. What challenges have you faced in managing your chronic pain?
4. How has chronic pain affected your relationships with family and friends?
5. In what ways has chronic pain affected your employment or educational opportunities?
## Probing questions
1. Can you provide more details about the emotional impact of living with chronic pain?
2. How has your support system influenced your ability to manage chronic pain?
3. In what ways have you had to adapt your lifestyle to accommodate your chronic pain?
## Wrap-up
Thank you for your insights and contributions to this discussion. Your experiences and perspectives will help us better understand the challenges faced by patients with chronic pain and inform future research and treatment approaches.
Remember, this is just an example. When designing your focus group guide, consider the specific research objectives, target population, and context of your study.
Data Collection Techniques
Data Cleaning and Management
Importance of Data Cleaning
Data cleaning, also known as data cleansing or data wrangling, is the process of identifying and correcting or removing inaccurate, incomplete, or irrelevant data from a dataset. Data cleaning is a crucial step in the data analysis process, as it helps to ensure that the data is accurate, consistent, and suitable for analysis. Without proper data cleaning, the results of any analysis may be misleading or inaccurate, making it difficult to draw meaningful conclusions from the data.
Common Data Cleaning Techniques
There are several common data cleaning techniques that are used to identify and correct errors in a dataset. These include:
- Outlier detection: This technique involves identifying and removing data points that are significantly different from the rest of the dataset. Outliers can be caused by errors in data entry, measurement errors, or other factors.
- Data transformation: This technique involves converting data from one format to another to make it more suitable for analysis. For example, converting data from text to numerical format or standardizing data to a common scale.
- Data imputation: This technique involves filling in missing data points with estimates or predictions based on the available data.
- Data filtering: This technique involves removing data points that are irrelevant or contain errors.
Data Management Tools
There are several data management tools that can help with the data cleaning process. These include:
- Excel: Excel is a popular tool for data cleaning and management. It includes several features, such as filters, pivot tables, and conditional formatting, that can help to identify and correct errors in a dataset.
- Python: Python is a programming language that is widely used for data analysis and cleaning. It includes several libraries, such as Pandas and NumPy, that can help to manipulate and clean data.
- R: R is another programming language that is commonly used for data analysis and cleaning. It includes several packages, such as dplyr and tidyr, that can help to manipulate and clean data.
By following proper data cleaning techniques and using appropriate data management tools, researchers can ensure that their data is accurate, consistent, and suitable for analysis.
Data Analysis Techniques
Data analysis techniques are methods used to examine and interpret data collected through various research methods. These techniques are used to extract meaningful insights from raw data, which can be used to answer research questions or support hypotheses. There are two main types of data analysis techniques: qualitative and quantitative.
Qualitative Data Analysis Techniques
Qualitative data analysis techniques are used to analyze non-numerical data, such as text, images, audio, and video. These techniques are used to identify patterns, themes, and meanings in the data. Some common qualitative data analysis techniques include:
- Content analysis: This technique involves analyzing the content of text, images, or other media to identify patterns or themes.
- Thematic analysis: This technique involves identifying patterns or themes in the data and categorizing them into themes.
- Discourse analysis: This technique involves analyzing language and communication patterns to identify underlying meanings and assumptions.
Quantitative Data Analysis Techniques
Quantitative data analysis techniques are used to analyze numerical data, such as survey responses, test scores, or demographic data. These techniques are used to identify relationships between variables and make predictions based on statistical models. Some common quantitative data analysis techniques include:
- Descriptive statistics: This technique involves summarizing and describing the characteristics of the data, such as mean, median, mode, and standard deviation.
- Inferential statistics: This technique involves making inferences about a population based on a sample of data, such as hypothesis testing and confidence intervals.
- Regression analysis: This technique involves identifying the relationship between two or more variables and making predictions based on that relationship.
Examples of Data Analysis Techniques
Here are some examples of data analysis techniques that can be used in different research contexts:
- Content analysis: A researcher might use content analysis to analyze social media posts to identify common themes or sentiment related to a particular topic.
- Thematic analysis: A researcher might use thematic analysis to identify patterns in qualitative interview data related to a particular research question.
- Descriptive statistics: A researcher might use descriptive statistics to summarize and describe the characteristics of a dataset, such as the average score on a test or the percentage of respondents who answered a particular question a certain way.
- Inferential statistics: A researcher might use inferential statistics to test a hypothesis about a population based on a sample of data, such as whether a particular treatment is more effective than a control group.
Overall, data analysis techniques are an essential part of the research process, as they allow researchers to extract meaningful insights from raw data and draw conclusions based on that data.
Ethical Considerations in Data Collection
Informed Consent
Explanation of Informed Consent
Informed consent is a critical aspect of data collection, particularly when working with human subjects. It is a process by which researchers obtain permission from participants to collect, use, and disseminate their data. Informed consent ensures that participants understand the purpose, procedures, risks, benefits, and their rights concerning the research. It is an essential ethical principle that protects the autonomy and privacy of research participants.
To obtain informed consent, researchers must provide potential participants with sufficient information about the study, such as the research objectives, methods, and expected outcomes. The information should be presented in a clear and concise manner, using language that is easily understandable by the participants. Researchers should also provide participants with the opportunity to ask questions and seek clarification before they make a decision to participate.
Example Informed Consent Form
An informed consent form is a document that outlines the key aspects of the study and the participant’s rights. It is typically signed by the participant to indicate their understanding and agreement to participate in the study. The form may include the following sections:
- Introduction: This section provides an overview of the study, including the research objectives, methods, and expected outcomes.
- Purpose: This section explains the purpose of the study and why it is important.
- Procedures: This section describes the procedures that participants will undergo, including any physical or psychological tests, interviews, or surveys.
- Risks and Benefits: This section outlines the potential risks and benefits of participating in the study. Researchers must disclose any foreseeable risks and explain how the benefits of the study outweigh the risks.
- Confidentiality and Privacy: This section explains how the data will be collected, stored, and disseminated. Researchers must assure participants that their personal information will be kept confidential and used only for the purposes of the study.
- Participant’s Rights: This section outlines the participant’s rights, including the right to withdraw from the study at any time, the right to access their data, and the right to receive appropriate compensation for their time and effort.
- Signature: This section includes a signature line for the participant to indicate their understanding and agreement to participate in the study.
In summary, informed consent is a critical aspect of data collection that ensures that participants understand the purpose, procedures, risks, benefits, and their rights concerning the research. It is an essential ethical principle that protects the autonomy and privacy of research participants. Researchers must provide potential participants with sufficient information about the study and obtain their informed consent before collecting their data.
Confidentiality and Privacy
Confidentiality and privacy are two critical ethical considerations in data collection. These considerations aim to protect the rights and interests of research participants while ensuring the accuracy and reliability of the data collected.
Explanation of Confidentiality and Privacy
Confidentiality refers to the obligation of researchers to protect the identities and personal information of research participants. Privacy, on the other hand, is the right of individuals to control access to their personal information.
Researchers must maintain confidentiality and respect the privacy of research participants throughout the data collection process. This includes protecting the personal information of participants, ensuring that data is collected and stored securely, and avoiding any potential harm to participants that may result from the disclosure of their personal information.
Measures to Ensure Confidentiality and Privacy
There are several measures that researchers can take to ensure confidentiality and privacy when collecting data. These include:
- Anonymizing data: Researchers can remove personal identifiers such as names, addresses, and contact details from data to protect the anonymity of participants.
- Using pseudonyms: Researchers can assign pseudonyms to participants to protect their identities while still allowing researchers to link data to specific individuals.
- Encrypting data: Researchers can encrypt data to protect it from unauthorized access and ensure that it is only accessible to authorized personnel.
- Secure storage: Researchers can store data securely, for example, by using password-protected files, limiting access to data, and storing data on secure servers.
- Informed consent: Researchers must obtain informed consent from participants before collecting data. Informed consent involves providing participants with detailed information about the research, including how their data will be collected, stored, and used.
By implementing these measures, researchers can ensure that they are upholding ethical standards and protecting the privacy and confidentiality of research participants.
Cultural Sensitivity
Cultural sensitivity refers to the awareness and respect for the cultural differences and values of individuals and groups in the data collection process. It is important to ensure that data collection methods and instruments do not offend or disadvantage particular cultural groups.
Measures to ensure cultural sensitivity in data collection include:
- Obtaining informed consent from participants, including an explanation of the purpose and potential risks of the study
- Ensuring that the data collection instrument is appropriate for the cultural context of the participants
- Providing an option for participants to withdraw from the study at any time
- Ensuring that the data collection process is transparent and avoids deception
- Avoiding the use of language or images that may be culturally insensitive or offensive
- Being mindful of power dynamics and avoiding exploitation or coercion of participants
- Providing an option for participants to remain anonymous if desired
- Avoiding generalizations or stereotypes based on cultural background
- Respecting the privacy and confidentiality of participants’ data
- Seeking advice from community members or cultural experts when necessary.
Data Quality
Explanation of Data Quality
Data quality refers to the overall value and integrity of the data collected. It encompasses various aspects, including accuracy, completeness, consistency, validity, and reliability. These factors collectively determine the usefulness and trustworthiness of the data. High-quality data is essential for making informed decisions, drawing meaningful conclusions, and ensuring the credibility of research findings.
Examples of Measures to Ensure Data Quality
To guarantee data quality, researchers employ a range of methods and techniques during the data collection process. Some of these measures include:
- Pilot testing: Conducting a small-scale trial run of the data collection instrument or technique helps identify any issues or problems that may arise. This allows researchers to refine and improve the process before it is implemented on a larger scale.
- Pre-testing: Before the actual data collection, researchers may pre-test the instrument or technique on a small sample to assess its effectiveness and to ensure that it is collecting the intended data. This also helps to identify any potential errors or biases that may need to be addressed.
- Standardization: To ensure consistency in data collection, researchers may use standardized procedures, such as clear and specific instructions for completing questionnaires or interviews. This helps to minimize variability in the data and enhance its reliability.
- Training: Providing training to data collectors ensures that they understand the data collection instrument or technique and can accurately and consistently collect the required data. This is particularly important when using complex instruments or techniques that require specialized knowledge or skills.
- Source documentation: Maintaining detailed records of the data collection process, including information about the instrument or technique used, the data collector, and any other relevant contextual information, helps to ensure the integrity and traceability of the data.
- Data validation: After data collection, researchers may conduct data validation checks to identify and correct any errors, inconsistencies, or outliers in the data. This helps to improve the accuracy and reliability of the data.
- Triangulation: By comparing and contrasting data from multiple sources or using different data collection techniques, researchers can enhance the validity and reliability of their findings. This helps to ensure that the data accurately reflects the phenomenon being studied.
By implementing these measures, researchers can improve the quality of the data they collect, ultimately strengthening the credibility and usefulness of their research findings.
Data Security
Explanation of Data Security
Data security refers to the protection of sensitive information from unauthorized access, use, disclosure, disruption, modification, or destruction. This involves implementing measures to safeguard the confidentiality, integrity, and availability of data. Data security is a critical concern for individuals, organizations, and governments alike, as it affects the privacy, trust, and reputation of those involved in data collection and processing.
Examples of Measures to Ensure Data Security
To ensure data security, researchers and organizations can implement various measures, including:
- Access control: Limiting access to data to only those who need it, based on their roles and responsibilities. This can be achieved through passwords, biometric authentication, or other verification methods.
- Encryption: Converting data into a coded format to prevent unauthorized access. This can be done using algorithms that scramble the data, making it unreadable without the appropriate key.
- Auditing and monitoring: Regularly reviewing and monitoring system access logs, network traffic, and data usage to detect any suspicious activities or breaches.
- Backup and recovery: Regularly creating backups of data and having a disaster recovery plan in place to ensure that data can be restored in case of loss or damage.
- Penetration testing: Simulating an attack on a system or network to identify vulnerabilities and weaknesses, and then addressing them before they can be exploited by real attackers.
- Compliance with regulations: Adhering to relevant laws and regulations, such as the General Data Protection Regulation (GDPR) or the Health Insurance Portability and Accountability Act (HIPAA), which impose specific requirements for data security and privacy.
- Security awareness training: Educating employees and stakeholders about the importance of data security, the risks associated with data breaches, and the steps they can take to protect sensitive information.
By implementing these measures, data collectors can minimize the risks associated with data security breaches and protect the confidentiality and integrity of the data they collect.
Avoiding Bias
Explanation of Bias
Bias in data collection refers to any systematic deviation from the truth or fairness in the data collection process. This can arise from various sources, including the researcher’s own beliefs, values, or assumptions, as well as from external factors such as social or cultural influences. Bias can have a significant impact on the validity and reliability of research findings, and it is essential to identify and mitigate bias in the data collection process.
Examples of Measures to Avoid Bias
- Sampling: Avoiding self-selection bias by using random sampling techniques, stratified sampling, or oversampling underrepresented groups.
- Instrument Design: Ensuring that questions are neutral, avoid loaded language, and provide adequate response options to prevent biased responses.
- Data Analysis: Using statistical techniques such as regression analysis, correlation analysis, or structural equation modeling to control for potential sources of bias in the data.
- Debiasing Techniques: Techniques such as the ‘Think Aloud’ protocol, where participants are asked to verbalize their thought processes while completing a task, can help reveal any underlying biases that may influence their responses.
- Triangulation: Using multiple sources of data, such as combining qualitative and quantitative methods, to cross-validate findings and ensure the credibility of the research.
- Researcher Reflexivity: Being aware of one’s own biases and actively working to minimize their impact on the research process, including data collection, analysis, and interpretation.
By taking these measures, researchers can significantly reduce the risk of bias in their data collection process, ensuring that their findings are valid, reliable, and trustworthy.
Other Ethical Considerations
While obtaining informed consent and protecting participant confidentiality are critical ethical considerations in data collection, there are other ethical issues that researchers must be aware of and address. This section discusses some of these additional ethical considerations and provides examples of measures to mitigate them.
- Privacy and Anonymity: Researchers must ensure that participants’ privacy and anonymity are protected, especially when collecting sensitive data. Techniques such as encrypting data, masking personal identifiers, and conducting data analysis in secure environments can help maintain privacy and anonymity.
- Voluntary Participation: Participants should be able to decide whether or not to participate in the study, and their decision should be respected. Researchers should not coerce or manipulate participants into participating, and should provide clear and accurate information about the study’s purpose, procedures, and potential risks or benefits.
- Deception: In some cases, researchers may need to deceive participants about the true nature of the study to obtain more accurate data. However, this practice should be used sparingly and only when necessary, and researchers must take steps to minimize harm and maintain trust with participants. For example, providing debriefing sessions or offering compensation for time and effort can help mitigate the potential negative effects of deception.
- Vulnerable Populations: Researchers must be cautious when working with vulnerable populations, such as children, the elderly, or individuals with mental or physical disabilities. These groups may be more susceptible to coercion or harm, and researchers must take extra precautions to protect their rights and welfare. This may involve obtaining additional consent from a guardian, providing additional support or accommodations, or working with trained professionals.
- Data Quality and Integrity: Researchers have a responsibility to ensure that the data they collect is accurate, reliable, and valid. This may involve using appropriate sampling techniques, selecting valid and reliable measurement instruments, and employing rigorous data analysis methods. Additionally, researchers should be transparent about their data collection and analysis procedures, and should document and preserve their data for future audits or reanalysis.
By being aware of these additional ethical considerations and taking appropriate measures to address them, researchers can ensure that their data collection practices are both ethical and effective.
FAQs
1. What are the different methods used to collect data?
There are several methods used to collect data, including surveys, interviews, observations, and experiments. Surveys involve asking a series of questions to a group of people to gather information about their attitudes, opinions, or behaviors. Interviews are a more in-depth method of data collection, where a researcher asks questions to an individual or a group to gain insight into a specific topic. Observations involve observing and recording behavior or phenomena in a natural setting. Experiments involve manipulating variables to determine cause-and-effect relationships.
2. What are the different instruments used to collect data?
There are various instruments used to collect data, including questionnaires, interviews, observations, and tests. Questionnaires are a type of instrument used to collect data through a series of questions. Interviews can be conducted in person, over the phone, or online, and involve a researcher asking questions to an individual or group. Observations involve using tools such as cameras, recorders, or field notes to observe and record behavior or phenomena. Tests are used to measure knowledge, skills, or abilities, and can include multiple-choice questions, essays, or practical tasks.
3. What are some techniques used to ensure data quality?
There are several techniques used to ensure data quality, including random sampling, triangulation, and member checking. Random sampling involves selecting a representative sample from a larger population to ensure that the data collected is representative of the population as a whole. Triangulation involves using multiple methods of data collection to confirm findings and ensure data accuracy. Member checking involves asking participants to review and verify the data collected to ensure it accurately reflects their experiences or opinions. Other techniques include using trained observers, pilot testing, and double-coding to ensure consistency in data collection.